Monte Carlo Simulation Definition
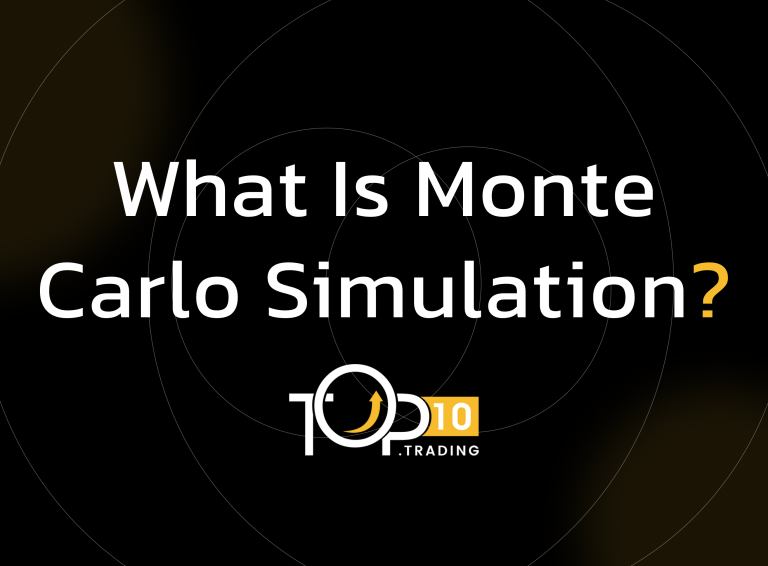
Monte Carlo Simulation is a statistical method used to model the probability of different outcomes in a process that involves random variables. In trading and investing, it helps assess risk, forecast potential returns, and test strategies by simulating a wide range of possible market scenarios based on historical data and assumed distributions.
Key Takeaways
- Monte Carlo Simulation runs thousands of random scenarios to estimate potential outcomes.
- It is used in finance to assess portfolio risk, pricing models, and trading strategies.
- The method relies on repeated random sampling to simulate market behavior.
- It offers a probabilistic view of future performance rather than a single prediction.
- Useful in both risk management and decision-making under uncertainty.
How Monte Carlo Simulation Works
The simulation generates a large number of potential future outcomes based on random inputs within defined parameters. For example, it might simulate 10,000 possible price paths for a stock or portfolio over the next year, using assumptions about volatility, returns, and correlations.
By analyzing the range and distribution of outcomes, investors can estimate the probability of gains, losses, or breakeven scenarios. It is particularly useful in modeling complex systems with multiple uncertain factors, such as options pricing, retirement planning, or portfolio stress testing.
Examples of Monte Carlo Simulation
- A portfolio manager runs simulations to estimate the likelihood of a portfolio dropping more than 10% in a year.
- An options trader uses the model to predict potential outcomes for a multi-leg strategy.
- A financial planner applies Monte Carlo Simulation to assess the success rate of a retirement savings plan under various market conditions.
Benefits of Monte Carlo Simulation
- Probabilistic Forecasting: Provides a range of outcomes with associated probabilities.
- Risk Insight: Helps visualize worst-case, best-case, and most likely scenarios.
- Strategy Testing: Evaluates how trading systems might perform under diverse conditions.
- Decision Support: Aids in making informed choices under uncertainty.
Costs and Limitations
- Data-Dependent: The quality of results depends on the accuracy of input assumptions.
- Complexity: Requires statistical and computational knowledge to implement correctly.
- Computational Intensity: Large simulations can be resource- and time-consuming.
- Not Predictive: It does not predict the future but offers statistical possibilities.
Who Uses Monte Carlo Simulation?
Monte Carlo Simulation is used by quantitative analysts, institutional investors, risk managers, actuaries, and even individual traders. It’s a go-to tool for modeling uncertainty in finance, engineering, insurance, and economics, especially when real-world experimentation is not practical or possible.